Making sense of data from Land Surface Models (LSMs)
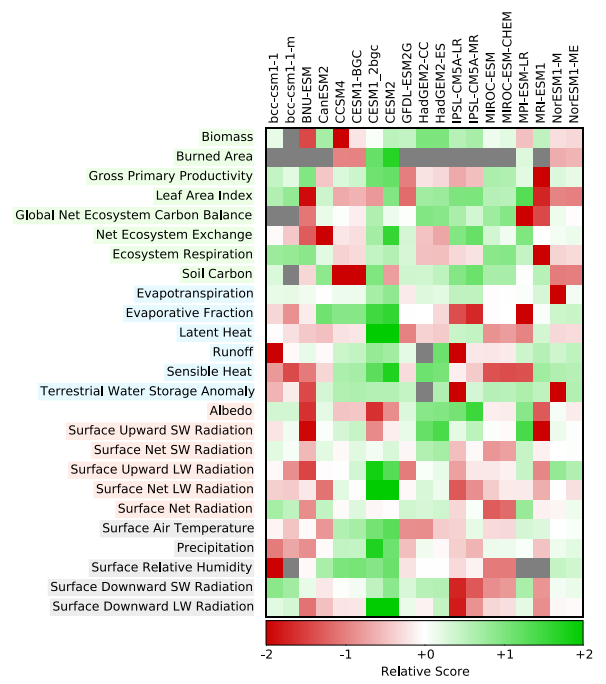
The land surface is a dynamic and complex component of the Earth system, shaping climate and biogeochemical cycles and serving as the origin of many anthropogenic impacts on the planet. Comprising complex and interactive organic and inorganic systems (soils, rocks, water in all phases, vegetation, and animals) the land surface shapes and is shaped by the fluid envelope of the Earth system on various timescales. The core objective of land surface models (LSMs) is to represent the physical and biogeochemical exchanges of energy, water, and other matter that comprise land-atmosphere interactions, especially under conditions of global change (Bonan, 2019).
At the same time, however, this objective has expanded to make terrestrial dynamics an object of inquiry, as reflected in land surface model development trends. LSMs increasingly represent ecosystem and human processes including ecosystem demographics, agriculture, urban areas, and their short- and long-term feedbacks to the Earth system. While LSMs were originally conceived as a boundary condition to constrain uncertainty in general circulation models, they have since become an essential Earth system modeling activity and locus of rapid model development. These ongoing advances have required and facilitated contact between the climate, hydrologic, ecosystem, agricultural, and land-use/land-cover science communities. Because LSMs integrate so many disciplines and processes, they are now applied in a wide variety of contexts (Blyth et al., 2021; Pitman, 2003).
Here we note the key processes and applications of LSMs as well as some analytical considerations for the responsible use of LSM data.
Expert User Guidance
The following was contributed by Corey Lesk and Justin Mankin, July 2023:
The land surface is a dynamic and complex component of the Earth system, shaping climate and biogeochemical cycles and serving as the origin of many anthropogenic impacts on the planet. Comprising complex and interactive organic and inorganic systems (soils, rocks, water in all phases, vegetation, and animals) the land surface shapes and is shaped by the fluid envelope of the Earth system on various timescales. The core objective of land surface models (LSMs) is to represent the physical and biogeochemical exchanges of energy, water, and other matter that comprise land-atmosphere interactions, especially under conditions of global change (Bonan, 2019).
At the same time, however, this objective has expanded to make terrestrial dynamics an object of inquiry, as reflected in land surface model development trends. LSMs increasingly represent ecosystem and human processes including ecosystem demographics, agriculture, urban areas, and their short- and long-term feedbacks to the Earth system. While LSMs were originally conceived as a boundary condition to constrain uncertainty in general circulation models, they have since become an essential Earth system modeling activity and locus of rapid model development. These ongoing advances have required and facilitated contact between the climate, hydrologic, ecosystem, agricultural, and land-use/land-cover science communities. Because LSMs integrate so many disciplines and processes, they are now applied in a wide variety of contexts (Blyth et al., 2021; Pitman, 2003).
Here we note the key processes and applications of LSMs as well as some analytical considerations for the responsible use of LSM data.
Key processes
Energy budget closure is the most essential process simulated by Earth system models. As such, a key priority for LSMs is the surface energy balance, through which absorbed solar and longwave radiation is balanced by outgoing longwave radiation, sensible and latent heat fluxes, and surface heat storage. Solving this balance requires LSMs to reckon not just for land surface heterogeneities, but with terrestrial hydrology and turbulent fluxes as well. This is why soil moisture and surface resistances to latent and sensible heat are such crucial features of LSMs.
In particular, the vegetated canopy influences surface resistance to latent heat flux largely through stomatal physiology, and provides the avenue by which the atmosphere accesses deep soil moisture. Contemporary LSMs now simulate this physiology directly as an optimization between carbon uptake by the land (photosynthesis) and water loss from it (transpiration and leaf evaporation), which depends on soil moisture and boundary layer atmospheric humidity. The demographic properties of vegetation, including plant functional types, phenology, and mortality, are increasingly simulated directly by LSMs, as they influence how vegetation affects the atmospheres. LSMs are thus an essential and dynamic convergence among the energy, water, and carbon cycles.
Soil moisture is determined by the surface water balance, in which precipitation less evapotranspiration accumulates in soils unless infiltration or saturation capacities are exceeded – at which point the excess runs off. Contemporary LSMs also account for ice and snow, vegetation dynamics, nutrient cycling, urban landforms, and their influences on water and energy balances (Blyth et al., 2021; Fisher & Koven, 2020). In contrast to their ocean and atmospheric counterparts, which use dynamical equations to resolve process dynamics at broad scales, land surface models rely almost entirely parameterization schemes to represent their dynamism. Importantly, these LSM physics have emerged as a key source of structural uncertainty in global change projections.
Applications of LSMs
LSMs are generally applied in three broad contexts: as a component of data assimilation systems, as parts of climate models (Earth system and general circulation models), and as experimental modeling tools. Depending on the goal, LSMs can be run in fully-coupled (with an interactive atmosphere to capture land-atmosphere interactions) or offline modes (i.e., forced by observed or idealized atmospheric evolution to isolate unidirectional atmospheric effects on land).
Many of the variables involved in the surface energy and water balances, such as total column soil moisture and transpiration, are difficult or currently impossible to directly and extensively observe. Spatiotemporally-complete fields of such variables can be inferred within a consistent physical framework by using offline LSMs driven by satellite- or ground-based weather observations (which are typically better observed). Examples of such datasets include the Global Land Data Assimilation System using the Noah, Variable Infiltration Capacity (VIC), and Catchment LSMs (Rodell et al., 2004) or the Community Land Model (CLM). Other surface reanalyses include ERA5-Land, which uses the H-TESSEL LSM (Muñoz-Sabater et al., 2021).
LSMs are now an essential component in the climate models largely relied upon for projections of future climate and carbon cycle change, climate attribution, and other applications. Climate models that include biogeochemically-active LSMs (and representations of other key Earth system components) are called Earth system models. For example, CLM version 5 (Lawrence et al., 2019) is included in the Community Earth System Model version 2 (CESM2) and the Norwegian Earth System Model version 2 (NorESM2), and the CLASS-CTEM LSM (Verseghy, 2000) is used in the Canadian Earth System Model version 5 (CanESM5). The inclusion of LSMs within ESMs enables them to represent key feedbacks within the Earth system, such as carbon cycle responses to warming (Humphrey et al., 2021; Trugman et al., 2018).
Finally, LSMs can be run in a variety of experimental configurations for basic research. Several LSM experiments were coordinated under the Coupled Model Intercomparison Project’s latest Phase 6 (CMIP6, Eyring et al., 2016), including the Land Surface, Snow, and Soil Moisture Model Intercomparison Project (LS3MIP, Van Den Hurk et al., 2016), Land Use Model Intercomparison Project (LUMIP, Lawrence et al., 2016), and the Coupled Climate-Carbon Cycle Model Intercomparison Project (C4MIP, Jones et al., 2016). Data are available from https://esgf-node.llnl.gov/search/cmip6/.These projects have generated insights into the effects of land-atmosphere coupling, vegetation, and land-use change on hydroclimate extremes and informed ongoing model development. For instance, LSM studies integrating historical crop cover and irrigation data have clarified the impact of human land use on land surface properties and thus climate (Singh et al., 2018).
Considerations when using LSM data
Several limitations of LSMs are important to keep in mind when applying LSM data. These limitations generally correspond to the key challenges and frontiers of development in land-surface modelling (Fisher & Koven, 2020).
1. LSMs are models, not observations. While LSM data are useful across disciplines, appropriate use of LSM data requires an understanding of their biases and awareness of the potential for errors (Ukkola et al., 2016). These data weaknesses can partly be ascertained through comparison against ground-based or satellite observations where they are available. Coordinated benchmarking procedures, such as the Internationally Land Model Benchmarking (ILAMB) system (Collier et al., 2018), have been developed to ease and harmonize this process, though it remains the case that LSMs are difficult to validate.
2. LSMs are mechanistically incomplete. Partial or lacking representation of key processes is part of why LSM data contain biases and inaccuracies. Many processes are simulated using parameters whose values are poorly constrained or highly variable (Kala et al., 2016). Certain processes, such as nutrient limitations on photosynthesis and agricultural management, are at the forefront of scientific understanding and model development, and can interact with each other. Enhancing the representation and evaluation of complex interactions among processes is a current priority in LSM development. Understanding which processes are represented in LSMs, and at what level of complexity, is important to effectively interpret and apply the data.
3. LSMs are run at set and variable spatiotemporal resolutions. As such, the level of specificity of the data in time or space are constrained by these resolutions (typically several kilometers or less, and over time scales ranging from hours to years). Because the land surface is particularly spatially heterogeneous (Torres-Rojas et al., 2022), specific locations may exhibit different land surface dynamics than the larger grid cell. Improving the representation of subgrid-scale heterogeneity is a priority for ongoing LSM development. In the interim, sound application of LSM data requires recognizing that their resolution may not be appropriate for every application.
LSMs are powerful tools to characterize and understand the land surface and its role in future global change. As they continue to develop rapidly, incorporating richer treatments of biogeophysics and biogeochemistry, data users should be aware that these choices will undoubtedly shape the simulation of larger atmospheric and oceanic responses and key feedbacks that determine global change. In light of the present limitations and uncertainties, careful and responsible use of LSM data should include and consider data from as many LSMs as possible. The use of multiple LSMs allows researchers to sample, if not understand, these uncertainties, and ensure that the state of land surface modeling is effectively represented in any broader application. #
Cite this page
Acknowledgement of any material taken from or knowledge gained from this page is appreciated:
Mankin, Justin &, Lesk, Corey & National Center for Atmospheric Research Staff (Eds). Last modified "The Climate Data Guide: Making sense of data from Land Surface Models (LSMs).” Retrieved from https://climatedataguide.ucar.edu/climate-data/making-sense-data-land-surface-models-lsms on 2024-07-26.
Citation of datasets is separate and should be done according to the data providers' instructions. If known to us, data citation instructions are given in the Data Access section, above.
Acknowledgement of the Climate Data Guide project is also appreciated:
Schneider, D. P., C. Deser, J. Fasullo, and K. E. Trenberth, 2013: Climate Data Guide Spurs Discovery and Understanding. Eos Trans. AGU, 94, 121–122, https://doi.org/10.1002/2013eo130001
Key Figures
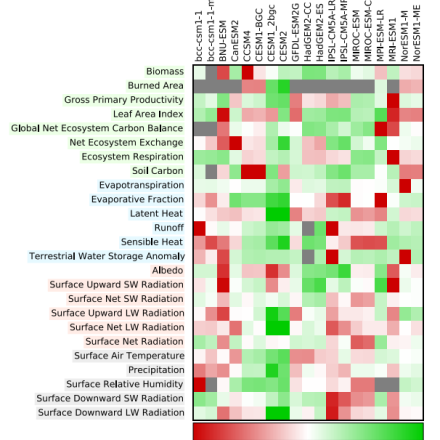
Figure 2: Simulation performance of 19 Earth system models (columns) across a range of land-surface variables (rows) based on the International Land Model Benchmarking (ILAMB) system. The relative score represents the performance of models relative to each other (absolute score is also available from ILAMB). Grey boxes indicate missing variables. Figure adapted from Collier et al. 2018. (credit: Justin Makin and Corey Lesk)
Other Information
- Blyth, E. M., and Coauthors, 2021: Advances in Land Surface Modelling. Curr Clim Change Rep, 7, 45–71, https://doi.org/10.1007/s40641-021-00171-5.
- Bonan, G. (2019). Climate Change and Terrestrial Ecosystem Modeling. Climate Change and Terrestrial Ecosystem Modeling, 1–438. https://doi.org/10.1017/9781107339217
- Collier, N., Hoffman, F. M., Lawrence, D. M., Keppel-Aleks, G., Koven, C. D., Riley, W. J., Mu, M., & Randerson, J. T. (2018). The International Land Model Benchmarking (ILAMB) System: Design, Theory, and Implementation. Journal of Advances in Modeling Ea
- Eyring, V., Bony, S., Meehl, G. A., Senior, C. A., Stevens, B., Stouffer, R. J., & Taylor, K. E. (2016). Overview of the Coupled Model Intercomparison Project Phase 6 (CMIP6) experimental design and organization. Geoscientific Model Development, 9(5), 193
- Fisher, R. A., & Koven, C. D. (2020). Perspectives on the Future of Land Surface Models and the Challenges of Representing Complex Terrestrial Systems. Journal of Advances in Modeling Earth Systems, 12(4). https://doi.org/10.1029/2018MS001453
- Humphrey, V., Berg, A., Ciais, P., Gentine, P., Jung, M., Reichstein, M., Seneviratne, S. I., & Frankenberg, C. (2021). Soil moisture–atmosphere feedback dominates land carbon uptake variability. Nature, 592(7852), 65–69. https://doi.org/10.1038/s41586-02
- Jones, C. D., Arora, V., Friedlingstein, P., Bopp, L., Brovkin, V., Dunne, J., Graven, H., Hoffman, F., Ilyina, T., John, J. G., Jung, M., Kawamiya, M., Koven, C., Pongratz, J., Raddatz, T., Randerson, J. T., & Zaehle, S. (2016). C4MIP-The Coupled Climate
- Kala, J., De Kauwe, M. G., Pitman, A. J., Medlyn, B. E., Wang, Y. P., Lorenz, R., & Perkins-Kirkpatrick, S. E. (2016). Impact of the representation of stomatal conductance on model projections of heatwave intensity. Scientific Reports, 6(January), 1–7. ht
- Lawrence, D. M., Fisher, R. A., Koven, C. D., Oleson, K. W., Swenson, S. C., Bonan, G., Collier, N., Ghimire, B., van Kampenhout, L., Kennedy, D., Kluzek, E., Lawrence, P. J., Li, F., Li, H., Lombardozzi, D., Riley, W. J., Sacks, W. J., Shi, M., Vertenste
- Lawrence, D. M., Hurtt, G. C., Arneth, A., Brovkin, V., Calvin, K. V., Jones, A. D., Jones, C. D., Lawrence, P. J., Noblet-Ducoudré, N. De, Pongratz, J., Seneviratne, S. I., & Shevliakova, E. (2016). The Land Use Model Intercomparison Project (LUMIP) cont
- Muñoz-Sabater, J., Dutra, E., Agustí-Panareda, A., Albergel, C., Arduini, G., Balsamo, G., Boussetta, S., Choulga, M., Harrigan, S., Hersbach, H., Martens, B., Miralles, D. G., Piles, M., Rodríguez-Fernández, N. J., Zsoter, E., Buontempo, C., & Thépaut, J
- Pitman, A. J. (2003). The evolution of, and revolution in, land surface schemes designed for climate models. International Journal of Climatology, 23(5), 479–510. https://doi.org/10.1002/joc.893
- Rodell, M., Houser, P. R., Jambor, U., Gottschalck, J., Mitchell, K., Meng, C. J., Arsenault, K., Cosgrove, B., Radakovich, J., Bosilovich, M., Entin, J. K., Walker, J. P., Lohmann, D., & Toll, D. (2004). The Global Land Data Assimilation System. Bulletin
- Singh, D., McDermid, S. P., Cook, B. I., Puma, M. J., Nazarenko, L., & Kelley, M. (2018). Distinct Influences of Land Cover and Land Management on Seasonal Climate. Journal of Geophysical Research: Atmospheres, 123(21), 12,017-12,039. https://doi.org/10.1
- Torres-Rojas, L., Vergopolan, N., Herman, J. D., & Chaney, N. W. (2022). Towards an Optimal Representation of Sub-Grid Heterogeneity in Land Surface Models. Water Resources Research, 58(12). https://doi.org/10.1029/2022WR032233
- Trugman, A. T., Medvigy, D., Mankin, J. S., & Anderegg, W. R. L. (2018). Soil Moisture Stress as a Major Driver of Carbon Cycle Uncertainty. Geophysical Research Letters, 45(13), 6495–6503. https://doi.org/10.1029/2018GL078131
- Ukkola, A. M., De Kauwe, M. G., Pitman, A. J., Best, M. J., Abramowitz, G., Haverd, V., Decker, M., & Haughton, N. (2016). Land surface models systematically overestimate the intensity, duration and magnitude of seasonal-scale evaporative droughts. Enviro
- Van Den Hurk, B., Kim, H., Krinner, G., Seneviratne, S. I., Derksen, C., Oki, T., Douville, H., Colin, J., Ducharne, A., Cheruy, F., Viovy, N., Puma, M. J., Wada, Y., Li, W., Jia, B., Alessandri, A., Lawrence, D. M., Weedon, G. P., Ellis, R., … Sheffield,
- Verseghy, D. L. (2000). The Canadian land surface scheme (CLASS): Its history and future. Atmosphere - Ocean, 38(1), 1–13. https://doi.org/10.1080/07055900.2000.9649637