Soil Moisture Data Sets: Overview & Comparison Tables
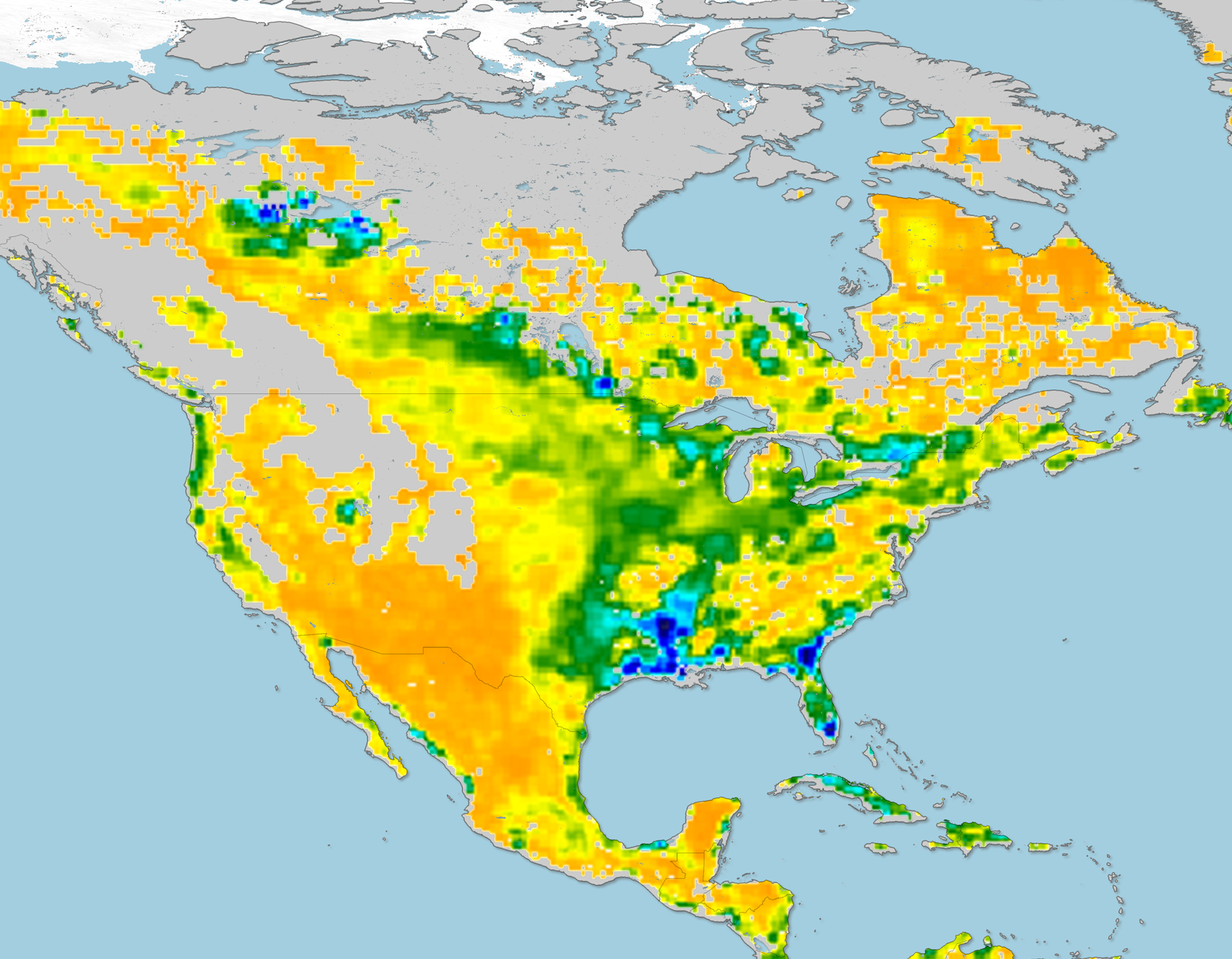
Soil moisture is a state variable of the land that crosses the interfaces of several disciplines, including meteorology, hydrology, climatology and ecology. Soil moisture content controls the partitioning of net radiation at the land surface between sensible and latent heat fluxes. This occurs primarily in the subtopics and tropics where rainfall is not abundant, and in the mid-latitudes during the warm season. Thus, accurate representation of soil moisture and its controls on surface fluxes are essential for water and energy cycle understanding and simulation, weather and climate forecasting (particularly at subseasonal to seasonal time scales) and the representation of changing feedbacks in a changing climate (Seneviratne et al. 2010; Dirmeyer et al. 2012, 2015).
Each type of soil moisture data set has its strengths and weaknesses, which are summarized in the table below. The best choice of product depends on the application. For instance, soil moisture for initializing a forecast model or Earth system model should be consistent with the statistics of the LSM in that model – it is never advisable to use a gridded soil moisture analysis as the soil moisture state in an LSM without first renormalizing the data in terms of standard deviations (anomalies) from the climatological annual cycle (Koster et al. 2009). Ideally, one should use a soil moisture analysis generated by the same LSM as used in the forecast model. Similarly, observational data should not be used to validate the absolute value of soil moisture in a model, as they do not necessarily correspond – model soil moisture is the result of soil moisture change calculated as the residual of the water balance (cf. Koster and Milly 1997). It is prudent to compare anomalies (in time) or spatial structures, and not RMSE or other 1st moment error statistics.
[The above text and table below were contributed by Dr. Paul Dirmeyer. Please see the Expert Guidance tab for the complete review and links to specific datasets.]
Strengths and Limitations of different types of soil moisture data sets | |||||||
---|---|---|---|---|---|---|---|
Category | Strengths | Limitations |
|||||
Ground observations |
|
|
|||||
Satellite observations |
|
• Gaps in coverage due to satellite orbits; complete coverage typically every ~8 days, but locally can be more frequent. • Prone to random and systematic errors • Microwave sensors only see surface (~3cm), do not see soil where vegetation is dense • Microwave sensors prone to radio interference, signals especially corrupted over urban areas • Gravity sensors have low spatial resolution, sense all water including water table fluctuations. |
|||||
Blended observations |
• Global coverage • Data are gridded • Fewer coverage gaps and can achieve higher temporal resolution than individual satellites |
• Time series inconsistencies can arise when different sensors come in/out of operation/inclusion. • Prone to random and systematic errors |
|||||
LSM-based analyses |
• Global coverage, no gaps in space or time • Data are gridded, include subsurface data • Somewhat constrained by observations (especially precipitation) • Not prone to random errors |
• Prone to systematic errors due to errors in model physics, soils or vegetation data sets used by models • Not direct or indirect observations – model characteristics will strongly flavor the soil moisture analyses • Most do not assimilate soil moisture observations • Likely not representative of variations at spatial scales smaller than model grid cell |
|||||
Reanalyses |
• Global coverage, no gaps in space or time • Data are gridded, include subsurface data |
• Prone to systematic errors due to errors in atmospheric and land model physics, soils and vegetation data sets used by models |
Expert User Guidance
The following was contributed by Dr. Paul Dirmeyer, Janurary 2019:
Long-Term Soil-Moisture Data Sets
Necessary Background Information
Soil moisture is a state variable of the land that crosses the interfaces of several disciplines, including meteorology, hydrology, climatology and ecology. Soil moisture content controls the partitioning of net radiation at the land surface between sensible and latent heat fluxes. This occurs primarily in the subtopics and tropics where rainfall is not abundant, and in the mid-latitudes during the warm season. Thus, accurate representation of soil moisture and its controls on surface fluxes are essential for water and energy cycle understanding and simulation, weather and climate forecasting (particularly at subseasonal to seasonal time scales) and the representation of changing feedbacks in a changing climate (Seneviratne et al. 2010; Dirmeyer et al. 2012, 2015).
Definitions of soil moisture vary, means of measuring it vary, even the scales over which it is defined vary. For example, the International Glossary of Hydrology defines "soil moisture" as the moisture contained in the portion of the soil which is above the water table. Contrast this to “soil water” which is defined as water suspended in the upper soil near the ground surface, that can be discharged into the atmosphere by evapotranspiration. The latter definition is closer to what land surface models (LSMs) represent, that are a part of most weather and climate models. There is a distinction between water that is readily available for evapotranspiration, accessible by plant roots, and water that is deeper below ground. Some models do simulate groundwater, but generally treat the region between the top couple meters of soil and the water table as a “black box” outside the model system.
Direct measurements of soil moisture are rare. There are only three methods of direct soil moisture measurement, all effectively weighing water below the land surface. Gravimetric measurements are labor intensive and falling out of favor – they involve digging a core of soil, typically down to ~1m depth, cutting it into layers, weighing each layer, baking the water out of each and reweighing. The difference in weight is the water content. A lysimeter also weighs soil to determine moisture, but does so in situ, with a container and scale buried into the ground. These two methods take very localized measurements. Water mass fluctuations are also deduced by small fluctuations in gravity measured from space (GRACE; Tapley et al. 2004). The last method cannot distinguish vertical structure, measuring variations in the water table as well as all water above it, and has a spatial granularity ≥100km.
Most soil moisture measurements are indirect, actually measuring properties correlated with soil moisture. In-ground sensors measure thermal, electrical (to measure soil dielectric constant), or radioactive (neutron) scattering to infer soil moisture content. Above ground, remote-sensing methods using microwave or thermal radiation bands, or cosmic rays are used – sensors may be near-field (a couple meters above the ground) flown aboard aircraft or satellites. The least expensive, and thus most common, soil moisture instruments are in-ground sensors that measure dielectric variations. Most soil moisture networks, typically deployed to serve agricultural interests, use dielectric sensors, although networks exist using each of the approaches listed here. There is no standardization like exists for official rain gauge networks, and direct coordination among networks is poor. Coverage is improving with time as the number and size of networks continues to grow.
Table of in situ and near-field instrument characteristics
Type |
Example Network |
Position |
Depth |
Scale |
Accuracy * |
Gravimetric |
RUSWET-AGRO |
In-ground sample |
0-1m |
Point |
<0.1 |
Dielectric (TDR) |
SCAN |
In-ground |
0.05-2m |
Point |
0.1-0.2 |
Dielectric (Capacitance) |
SOILSCAPE |
In-ground |
0.05-1m |
Point |
0.2 |
Neutron Probe (Active) |
Illinois State Water Survey (prior to 2008) |
In-ground |
0.1-2m |
0.2 m2 |
0.1 |
Cosmic Ray Neutron (Passive) |
COSMOS |
Above ground |
0.05-0.5m † |
0.1-1 km2 |
0.3 |
Heat Dissipation |
Oklahoma Mesonet |
In-ground |
0.05-1m |
Point |
0.1 |
GPS Reflection |
PBO-H2O |
Above ground |
≤0.05m † |
300m2 |
0.35 |
*Defined as where is the error variance and is the total variance of daily measurements (Dirmeyer et al. 2016). †Varies with soil type, moisture content
Ground networks offer spotty coverage and inter-network inconsistencies. Polar-orbiting satellites provide global coverage and consistency but have their own limitations. Satellite measurements of soil moisture based on microwave sensors are representative of only the top few centimeters of soil, and where vegetation cover is moderate to dense, they are not a measure of soil water at all. Over vegetation, water in and on the canopy is being measured. Until recently, most satellite measurements were collected from instruments designed for other purposes that happened to be sensitive to variations in soil moisture. These are chiefly from passive microwave-based sensors (AMSR-E, SMMR, TRMM, WindSat) and scatterometers (ERS-SCAT, METOP-ASCAT). Recently, two satellite missions have been launched with the specific purpose of measuring soil moisture, the Soil Moisture Ocean Salinity (SMOS; Kerr et al. 2010) and Soil Moisture Active/Passive (SMAP; Entekhabi et al. 2010) missions.
The most complete, internally consistent and easy-to-use gridded data sets of soil moisture are also the least connected to observations. They are the product of regional or global land surface models (LSMs) of varying levels of complexity, either run “offline” driven by observationally-based atmospheric forcing (e.g., from a combination of global gridded observational data and atmospheric reanalyses) or coupled to an atmospheric model (e.g., data from the reanalyses themselves). When the modeling system includes data assimilation, as with reanalyses, the results are more representative of observed conditions than models run in “open loop” climate modes. The offline LSM approach, pioneered by the Global Soil Wetness Project (Dirmeyer et al. 1999, 2006), is extensively used in LDAS (Land Data Assimilation System) products, even though they historically have not included assimilation or actual data. The most important input to an LSM for soil moisture simulation is, rather obviously, precipitation – so the quality of the soil moisture analysis is strongly tied to the quality of the input precipitation, and to a lesser extent radiation and near-surface meteorology.
Data sets
For ground-based measurements, the reader is referred to these two “networks of networks” that have compiled, homogenized and conducted quality control on diverse data sets, making them much easier to access and use for the research community.
The International Soil Moisture Network (ISMN; https://ismn.geo.tuwien.ac.at/) is a global network of networks. It was conceived as a database to provide ground-truth for satellite products, particularly SMOS. ISMN provides raw data from many in situ networks arranged by continent, but minimal processing beyond standardization of data formats and metadata. The ISMN data set includes precipitation, temperature (soil, surface and near surface air), and snow measurements where available. All data from every site in each network is archived, including multiple sensors and sensor replacements stored in separate ASCII files so all information is visible to the user. No gap-filling is applied to missing data. Detailed QC flags are included, and methodologies are well documented on the website.
The National Soil Moisture Network (NSMN; http://nationalsoilmoisture.com/), formerly the North American Soil Moisture Database (NASMD; Quiring et al. 2016), is limited to the United States, but contains more networks than ISMN, particularly state-level networks and mesonets. NSMN is working towards inter-network homogenization to produce a consistent observational product using high-resolution soils and precipitation data. Station having multiple sensors are blended into a single time series, and gap-filling is applied when gaps are not to large. Only soil moisture data are presented without ancillary variables. The result is more complete than ISMN but with less metadata provided and more geographically limited.
Most satellite data sets contain some form of mask or QC flags to indicate reliability of the data and whether soil is likely obscured by vegetation or other features (snow cover, urbanization, etc.). The one exception, as described above, is the GRACE data set, which represents a vertically-integrated water total. SMAP has demonstrably the highest information content of any satellite product (Kumar et al. 2018) and validates exceptionally well with in situ measurements (Colliander et al. 2017) – not surprising since it is the most modern. All satellite products are global.
Table of satellite products
Name |
Type |
Period |
H-Res |
T-Res |
Products |
Link |
SMAP |
µwave |
4/15-- |
9,36km |
Daily |
L1,2,3,4 |
|
SMOS |
µwave |
12/09-- |
50km |
Daily |
L0,1,2,3,4 |
|
AMSR-E |
µwave |
6/02-9/11 |
25km |
Daily |
L3 |
|
AMSR2 |
µwave |
5/12-- |
10, 25km |
Daily |
L2(NRT),3 |
|
TMI |
µwave |
12/97-04/15 |
25km |
Daily |
L3 |
|
SMM/I |
µwave |
7/87-- |
25km |
Daily |
L2 |
SMM/I |
WindSat |
µwave |
2/03-7/12 |
25km |
Daily |
L3 |
|
ASCAT |
Scatter. |
1/07-12/16 |
0.1° |
Monthly |
L3 |
ASCAT |
GRACE |
Gravity |
4/02—1/17 |
1° |
Monthly |
L3 |
There are hybrid / blended products that combine multiple sources of soil moisture information in an attempt to produce consistent data sets over long durations with minimal gaps in space and time. These are gridded products amenable to use for model comparison. The ESA CCI soil moisture project (https://www.esa-soilmoisture-cci.org/) blends scatterometer and microwave remote sensing products with versions using only active or passive instruments available. Data coverage spans 1978-2010 (when the ERS2 scatterometer data ended) daily at ¼° resolution. NOAA has begun a Soil Moisture Products System (SMOPS; https://data.nodc.noaa.gov/cgi-bin/iso?id=gov.noaa.ncdc:C00994) effort to create daily blended analyses using AMSR2, SMOS, ASCAT and SMAP data at ¼°. SMOPS data begin in March 2017.
Several LSM-based global soil moisture analyses are available, which are by definition “level 4”. The longest is the CPC soil moisture analysis (https://www.esrl.noaa.gov/psd/data/gridded/data.cpcsoil.html) with global data covering the period 1948-present at 0.5° resolution. It uses a very simple “leaky bucket” model driven by gridded monthly NCEP/NCAR reanalysis data and does not consider basic spatial variations in soil texture, orography, or spatial and temporal variations in vegetation, nor human-induced effects like irrigation. Fan and van den Dool (2004) state that the data should be used only as a “sanity check”.
Soil moisture analyses at higher spatial and temporal resolutions based on more modern LSMs are available. GLDAS (Rodell et al. 2004; https://ldas.gsfc.nasa.gov/gldas/) provides global analyses at 3-hourly, daily and monthly intervals at either 0.25° or 1° spatial resolution, depending on the version and LSM. Multiple LSMs are used, but no multi-model product is served, which would likely outperform individual model estimates for most applications (Guo et al. 2007). Forcing data sets for the LSMs are multiple, combining reanalyses, satellite and some rain gauge data in ways that are sometimes inconsistent in time, which can produce odd time series in some data sets – check documentation for specific products for caveats.
There are also offshoots of reanalyses that provide global soil moisture data. In MERRA2-Land (Reichle et al. 2017c) the Catchment LSM used in MERRA2 is re-run with the GCM precipitation replaced by a blended observationally-based precipitation analysis. ERA-Interim/Land uses almost the same process to produce an improved analysis of soil moisture for the ERA-Interim period 1979-2010 (Balsamo et al. 2015; data: https://apps.ecmwf.int/datasets/data/interim-land/type=an/). ERA5 will also have an “ERA5-Land” product, which will exceed ERA-5 spatial resolution; production is being led by Joaquín Muñoz-Sabater. The Level 4 SMAP product is an assimilation of SMAP retrievals into the Catchment LSM, and thus represents data assimilation and the influence of SMAP surface measurements on subsurface soil moisture, which is included in the product (Reichle et al. 2017a,b; data: https://gmao.gsfc.nasa.gov/GMAO_products/SMAP_L4/).
An analogous process was used to produce the NCEP Climate Forecast System Reanalysis (CFSR; Saha et al. 2010), which used a semi-coupled data assimilation where the Noah LSM was run offline driven by observationally-based meteorological analysis. States from that simulation were substituted into the reanalysis cycle once a day to prevent land states from drifting. Thus, CFSR, like the products described above, has a soil moisture analysis that is somewhat more constrained by observations, especially precipitation, than a typical reanalysis. Otherwise, reanalyses have not ingested soil moisture data directly as part of their data assimilation schemes until ERA5, which does assimilate ASCAT scatterometer data. Work is underway at ECMWF to assimilate SMOS in the operational analyses, which is expected to become operational in 2019.
Summary
Each type of soil moisture data set has its strengths and weaknesses, which are summarized in the table above. The figure, from (Kumar et al. 2018), shows the information content of some of the soil moisture products discussed here, shown as the density of grid cells mapped as a function of two statistics. Metric entropy (x-axis) is 0.0 for a constant field, 1.0 for a purely random time series, and ~0.5 for maximum information content. Fluctuation complexity (y-axis) indicates whether the sequence of values represents a simple (low) or complex (high) ordering of values. The satellite products (AMSR-E, ASCAT, SMOS, AMSR2) show a great deal of noise (high entropy, low complexity) except SMAP is somewhat less noisy and more informative. SCAN represents in situ measurements, closest to truth but still containing instrument errors. The two model products (GLDAS-Noah and GLDAS-Mosaic) tend to be less variable than SCAN observations, which probably reflects a combination of the LSMs being a bit simplistic, the observations containing random error, and the spatial scale difference between point measurements and coarse models.
The best choice of product depends on the application. For instance, soil moisture for initializing a forecast model or Earth system model should be consistent with the statistics of the LSM in that model – it is never advisable to use a gridded soil moisture analysis as the soil moisture state in an LSM without first renormalizing the data in terms of standard deviations (anomalies) from the climatological annual cycle (Koster et al. 2009). Ideally, one should use a soil moisture analysis generated by the same LSM as used in the forecast model. Similarly, observational data should not be used to validate the absolute value of soil moisture in a model, as they do not necessarily correspond – model soil moisture is the result of soil moisture change calculated as the residual of the water balance (cf. Koster and Milly 1997). It is prudent to compare anomalies (in time) or spatial structures, and not RMSE or other 1st moment error statistics.
A useful tool is being developed and maintained for validation and quality control of soul moisture using observational products by the Quality Assurance for Soil Moisture (QA4SM) project (https://qa4sm.eodc.eu/). ##
Cite this page
Acknowledgement of any material taken from or knowledge gained from this page is appreciated:
Dirmeyer, Paul & National Center for Atmospheric Research Staff (Eds). Last modified "The Climate Data Guide: Soil Moisture Data Sets: Overview & Comparison Tables.” Retrieved from https://climatedataguide.ucar.edu/climate-data/soil-moisture-data-sets-overview-comparison-tables on 2025-02-21.
Citation of datasets is separate and should be done according to the data providers' instructions. If known to us, data citation instructions are given in the Data Access section, above.
Acknowledgement of the Climate Data Guide project is also appreciated:
Schneider, D. P., C. Deser, J. Fasullo, and K. E. Trenberth, 2013: Climate Data Guide Spurs Discovery and Understanding. Eos Trans. AGU, 94, 121–122, https://doi.org/10.1002/2013eo130001
Key Figures
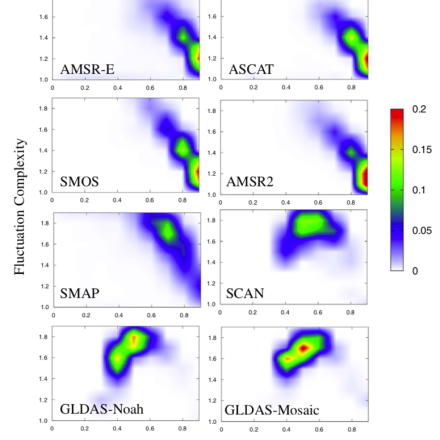
. The figure, from (Kumar et al. 2018), shows the information content of some of the soil moisture products discussed here, shown as the density of grid cells mapped as a function of two statistics. Metric entropy (x-axis) is 0.0 for a constant field, 1.0 for a purely random time series, and ~0.5 for maximum information content. Fluctuation complexity (y-axis) indicates whether the sequence of values represents a simple (low) or complex (high) ordering of values. The satellite products (AMSR-E, ASCAT, SMOS, AMSR2) show a great deal of noise (high entropy, low complexity) except SMAP is somewhat less noisy and more informative. SCAN represents in situ measurements, closest to truth but still containing instrument errors. The two model products (GLDAS-Noah and GLDAS-Mosaic) tend to be less variable than SCAN observations, which probably reflects a combination of the LSMs being a bit simplistic, the observations containing random error, and the spatial scale difference between point measurements and coarse model
Other Information
- Balsamo, G., and Coauthors, 2015: ERA-Interim/Land: a global land surface reanalysis data set. Hydrology and Earth System Sciences, 19, 389–407, doi:10.5194/hess-19-389-2015.
- Colliander, A., and Coauthors, 2017: Validation of SMAP surface soil moisture products with core validation sites. Remote Sensing of Environment, 191, 215–231, doi:10.1016/j.rse.2017.01.021
- Dirmeyer, P. A., A. J. Dolman, and N. Sato, 1999: The Pilot Phase of the Global Soil Wetness Project. Bulletin of the American Meteorological Society, 80, 851–878, doi:10.1175/1520-0477(1999)080<0851:tppotg>2.0.co;2.
- Dirmeyer, P. A., X. Gao, M. Zhao, Z. Guo, T. Oki, and N. Hanasaki, 2006: GSWP-2: Multimodel Analysis and Implications for Our Perception of the Land Surface. Bulletin of the American Meteorological Society, 87, 1381–1398, doi:10.1175/bams-87-10-1381.
- Dirmeyer, P. A., and Coauthors, 2012: Evidence for Enhanced Land–Atmosphere Feedback in a Warming Climate. Journal of Hydrometeorology, 13, 981–995, doi:10.1175/jhm-d-11-0104.1.
- Dirmeyer, P. A., G. Balsamo, and C. D. Peters-Lidard, 2015: Land-Atmosphere Interactions and the Water Cycle. Seamless Prediction of the Earth System: from Minutes to Months (G Brunet, S Jones, PM Ruti Eds.), WMO, World Meteorological Organization, Geneva
- Dirmeyer, P. A., and Coauthors, 2016: Confronting Weather and Climate Models with Observational Data from Soil Moisture Networks over the United States. Journal of Hydrometeorology, 17, 1049–1067, doi:10.1175/jhm-d-15-0196.1.
- Entekhabi, D., and Coauthors, 2010: The Soil Moisture Active Passive (SMAP) Mission. Proceedings of the IEEE, 98, 704–716, doi:10.1109/jproc.2010.2043918.
- Fan, Y., 2004: Climate Prediction Center global monthly soil moisture data set at 0.5° resolution for 1948 to present. Journal of Geophysical Research, 109, doi:10.1029/2003jd004345.
- Guo, Z., P. A. Dirmeyer, X. Gao, and M. Zhao, 2007: Improving the quality of simulated soil moisture with a multi-model ensemble approach. Quarterly Journal of the Royal Meteorological Society, 133, 731–747, doi:10.1002/qj.48.
- Kerr, Y. H., and Coauthors, 2010: The SMOS Mission: New Tool for Monitoring Key Elements ofthe Global Water Cycle. Proceedings of the IEEE, 98, 666–687, doi:10.1109/jproc.2010.2043032.
- Koster, R. D., and P. C. D. Milly, 1997: The Interplay between Transpiration and Runoff Formulations in Land Surface Schemes Used with Atmospheric Models. Journal of Climate, 10, 1578–1591, doi:10.1175/1520-0442(1997)010<1578:tibtar>2.0.co;2.
- Koster, R. D., Z. Guo, R. Yang, P. A. Dirmeyer, K. Mitchell, and M. J. Puma, 2009: On the Nature of Soil Moisture in Land Surface Models. Journal of Climate, 22, 4322–4335, doi:10.1175/2009jcli2832.1.
- Kumar, S. V., P. A. Dirmeyer, C. D. Peters-Lidard, R. Bindlish, and J. Bolten, 2018: Information theoretic evaluation of satellite soil moisture retrievals. Remote Sensing of Environment, 204, 392–400, doi:10.1016/j.rse.2017.10.016.
- Quiring, S. M., T. W. Ford, J. K. Wang, A. Khong, E. Harris, T. Lindgren, D. W. Goldberg, and Z. Li, 2016: The North American Soil Moisture Database: Development and Applications. Bulletin of the American Meteorological Society, 97, 1441–1459, doi:10.1175
- Reichle, R. H., and Coauthors, 2017: Assessment of the SMAP Level-4 Surface and Root-Zone Soil Moisture Product Using In Situ Measurements. Journal of Hydrometeorology, 18, 2621–2645, doi:10.1175/jhm-d-17-0063.1.
- Reichle, R. H., and Coauthors, 2017: Global Assessment of the SMAP Level-4 Surface and Root-Zone Soil Moisture Product Using Assimilation Diagnostics. Journal of Hydrometeorology, 18, 3217–3237, doi:10.1175/jhm-d-17-0130.1.
- Reichle, R. H., Q. Liu, R. D. Koster, C. S. Draper, S. P. P. Mahanama, and G. S. Partyka, 2017: Land Surface Precipitation in MERRA-2. Journal of Climate, 30, 1643–1664, doi:10.1175/jcli-d-16-0570.1.
- Rodell, M., and Coauthors, 2004: The Global Land Data Assimilation System. Bulletin of the American Meteorological Society, 85, 381–394, doi:10.1175/bams-85-3-381
- Saha, S., and Coauthors, 2010: The NCEP Climate Forecast System Reanalysis. Bulletin of the American Meteorological Society, 91, 1015–1058, doi:10.1175/2010bams3001.1.
- Seneviratne, S. I., T. Corti, E. L. Davin, M. Hirschi, E. B. Jaeger, I. Lehner, B. Orlowsky, and A. J. Teuling, 2010: Investigating soil moisture–climate interactions in a changing climate: A review. Earth-Science Reviews, 99, 125–161, doi:10.1016/j.earsc
- Tapley, B. D., 2004: GRACE Measurements of Mass Variability in the Earth System. Science, 305, 503–505, doi:10.1126/science.1099192.