GRACE: Gravity Recovery and Climate Experiment: Surface mass, total water storage, and derived variables
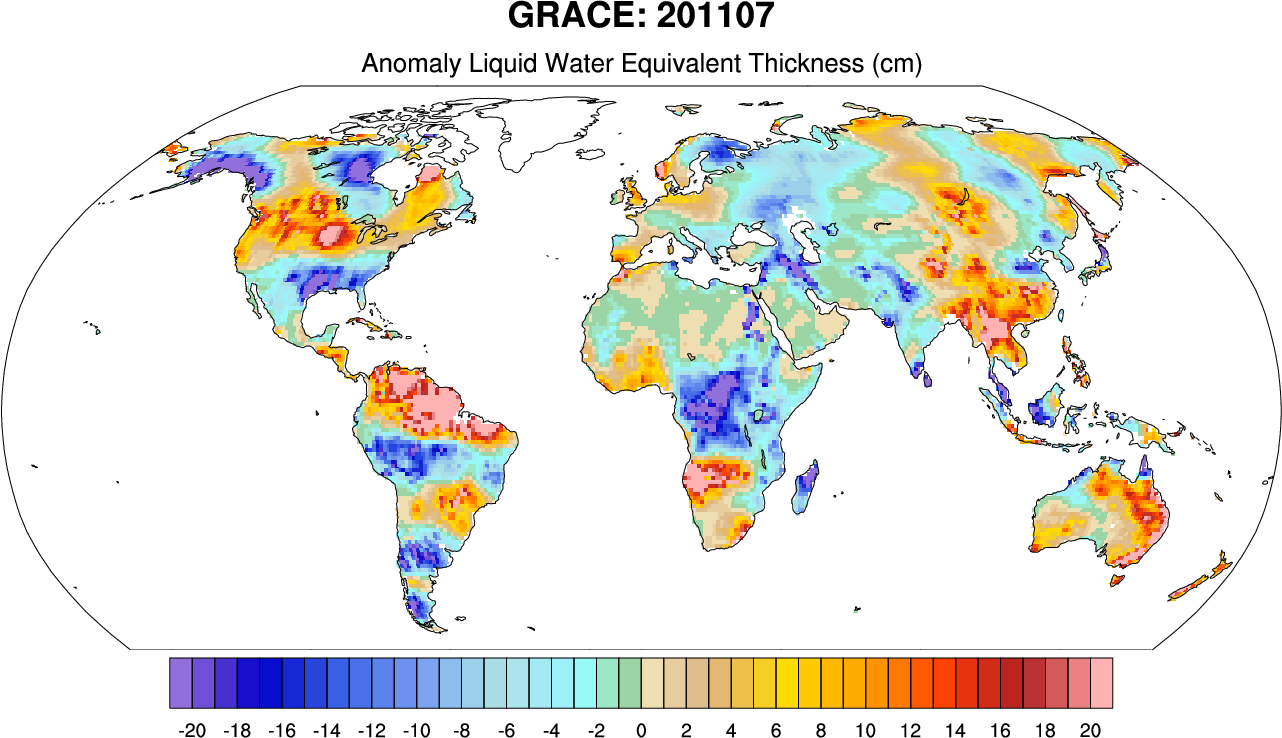
The Gravity Recovery and Climate Experiment (GRACE) refers to a pair of NASA satellites that has flown in low-Earth orbit since 2002. The satellites use a precise microwave ranging system to measure the distance between themselves due to gravitational acceleration. Onboard GPS instruments determine the exact position of the satellites over the Earth. GRACE measures changes in Earth's gravity field, which are directly related to changes in surface mass. The surface mass signal largely reflects total water storage (TWS); over the ocean TWS is interpreted as ocean bottom pressure and on land it is the the sum of groundwater, soil moisture, surface water, snow and ice. On longer timescales, GRACE measurements can reflect post-glacial rebound. Large earthquakes can also lead to mass changes and anomalies in the gravity measurements. GRACE measurements have also been used to examine changes in the extremes of the water cycle (more intense drought and rainfall) with climate warming.
Key Strengths
Relies on robust relationship between gravity and surface mass, rather than empirical paramaterizations and calibrations with in-situ measurements
Used to track volume and movement of water through reservoirs of the hydrologic cycle
Key Limitations
Coarse spatial and temporal resolutions
The raw data require filtering to be interpretable, and the nature and effects of the various filtering strategies can be difficult to trace
Expert User Guidance
#The following was contributed by Sean C. Swenson (NCAR), May, 2012:
In your view, what are the key strengths and key weaknesses of this data set?
Strengths: GRACE measures changes in the Earth's gravity field, which at monthly timescales, are related to changes in surface mass. Whereas some radiometric remote sensing products (e.g. precipication, snow water equivalent, soil moisture) are based on empirical parameterizations that require calibration with in situ observations, the relationship between the gravity field and surface mass used by GRACE is considered fairly robust, it is called Newton's Law of Gravity. Because GRACE senses changes in surface mass, it can be used to infer changes in land ice, land water storage, and ocean bottom pressure.
Weaknesses: The spatial and temporal resolution of the GRACE dataset are relatively coarse, i.e. pixel sizes of roughly 300-400 km at monthly intervals. The raw gravity field data are typically in the form of spherical harmonic coefficients, which contain measurement errors that are non-uniform. To obtain a useful combination of resolution and accuracy, the data must be filtered (or regularized in a least squares sense, which is a form of filtering). The number of filtering strategies, and the lack of information on the filtering method's effect on the signal, can be confusing.
What analyses have you used these data for?
GRACE data have been used to estimate changes in land ice (e.g. ice caps and mountain glaciers), winter snow accumulation, groundwater withdrawal, and model validation. These data are being included in land data assimilation schemes and drought monitoring products. GRACE has been used to estimate global ocean mass changes. Mass changes associated with large earthquakes can also be observed with GRACE.
What are some of the strategies for making optimal use of these data?
GRACE is sensitive to *all* mass changes, and thus measures the vertically integrated change in mass. To use GRACE to constrain a subset of the vertical mass budget, e.g. snow or groundwater, one must use independent data to remove the other components of the mass budget. Filtering the data is an important step in reducing measurement error, but it comes at the expense of signal modification. Performing simulations in which the data processing methods are applied to synthetic datasets provides valuable information on the effects of filtering and regularization.
What are the strategies for comparing these data with models?
The two main ways to equitably compare GRACE to model data are 1) process the model data in the same manner asthe GRACE data, i.e. filter the model using the GRACE filtering method; and 2) estimate the signal modification via simulations and restore any power lost due to filtering. The latter method is described in Landerer and Swenson (2012) - Key Publication #3 below.
What are some of the common pitfalls or mistakes people encounter when using these data?
The main mistakes that have been made are 1) comparing data of different spatial resultion, e.g. using GRACE to estimate total water storage (TWS) in a very small region and comparing to a fine-scale model or in situ data. In this case, GRACE does not adequately resolve small regions, and the comparison suffers from a difference in spatial scale akin to comparing satellite precipitation to a rain gage; 2) failing to adequately separate the GRACE signal. For example, if one wishes to use GRACE to estimate groundwater changes, one must first remove all other signals, i.e. soil moisture and surface water. Any errors in the subtracted signals will be present in the residual GRACE groundwater signal.
What are some comparable datasets,if any?
There are a handful of different groups having the expertise to generate gravity field data from the GRACE instrument data, and each dataset is somewhat different depending on the choices made during the data processing. Perhaps the biggest differences are apparent in the "constrained" or regularized solutions, in which the filtering is applied during the gravity field determination step. These datasets contain much less measurement error, and appear quite clean. However, regularization is simply a form of filtering, and signal modification occurs to some degree. The downside to constrained solutions is that the end-user cannot estimate the possible signal modification, and therefore biases resulting from regularization cannot be adequately quantified. This results in an underestimation of errors in the data.##
Cite this page
Acknowledgement of any material taken from or knowledge gained from this page is appreciated:
Swenson, Sean & National Center for Atmospheric Research Staff (Eds). Last modified "The Climate Data Guide: GRACE: Gravity Recovery and Climate Experiment: Surface mass, total water storage, and derived variables.” Retrieved from https://climatedataguide.ucar.edu/climate-data/grace-gravity-recovery-and-climate-experiment-surface-mass-total-water-storage-and on 2025-07-02.
Citation of datasets is separate and should be done according to the data providers' instructions. If known to us, data citation instructions are given in the Data Access section, above.
Acknowledgement of the Climate Data Guide project is also appreciated:
Schneider, D. P., C. Deser, J. Fasullo, and K. E. Trenberth, 2013: Climate Data Guide Spurs Discovery and Understanding. Eos Trans. AGU, 94, 121–122, https://doi.org/10.1002/2013eo130001
Key Figures
Other Information
approx. 300-400 km
- Tapley, B.D., S. Bettadpur, M. Watkins, and C. Reigber (2004). The gravity recovery and climate experiment: Mission overview and early results. Geophysical Research Letters, 31, L09607
- Swenson, S., J. Wahr, and P.C.D. Milly (2003), Estimated accuracies of regional water storage variations inferred from the Gravity Recovery and Climate Experiment (GRACE), Water Resources Research, 39 (8), 1223
- Landerer, F. W. and S. C. Swenson (2012), Accuracy of scaled GRACE terrestrial water storage estimates, Water Resour. Res., doi:10.1029/2011WR011453
- GRACE Publications list - NASA JPL
- GRACE Publications - U Texas
- Rodell, M., and B. Li, 2023: Changing intensity of hydroclimatic extreme events revealed by GRACE and GRACE-FO. Nat Water, 1, 241–248, https://doi.org/10.1038/s44221-023-00040-5.