GHCN-D: Global Historical climatology Network daily temperatures
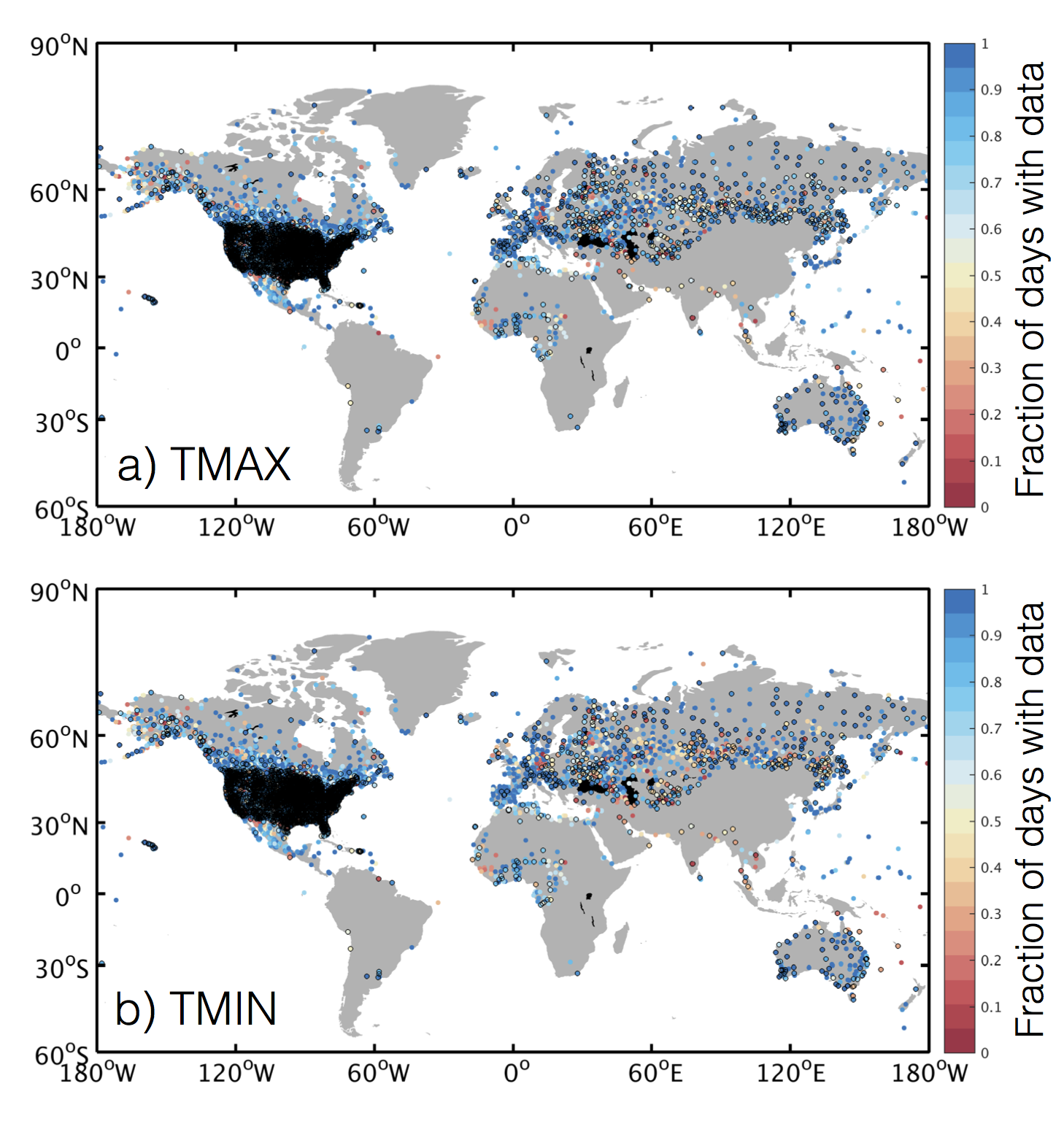
The Global Historical Climatology Network Daily database, GHCN-D, contains meteorological measurements from over 90,000 stations across the globe. The majority of station records contain precipitation data only, however other key variables including maximum temperature, minimum temperature, snowfall, cloudiness, wind speed and snow depth are available at many locations. This overview focuses on the temperature data. This is a key dataset for the validation of temperature estimates from satellites and atmospheric reanalyses. In addition, the numerous long records in the database are useful for studies of changes in mean temperatures and extremes. Users should be aware of the many sources of inhomogeneities in the records, as reviewed in the 'Expert Guidance' section of this page.
Researchers focusing on the continental United States may be interested in NOAA's nCLIMGRID, a 5-km gridded version of GHCN-D, available on the monthly timestep for 1895-present and daily for 1951-present. A related monthly dataset, nCLIMDIV, aggregates the data to the climate division and state levels and is used for NOAA's climate monitoring (see the 'Data Access' section for links). E-OBS provides comparable gridded data for Europe. Users seeking very high-resolution daily data for North America may also be interested in Daymet or PRISM (see "Related pages" sidebar for links).
Key Strengths
Direct measurements of surface temperature with no interpolation or model assumptions
Contains many long-term individual station records
Key Limitations
Unequal spatial coverage
Records contain many inhomogeneities owing to shifts in observation time, station location, and type of thermometer used
Expert User Guidance
The following was contributed by Karen McKinnon, February, 2016:
General overview
The Global Historical Climatology Network-Daily Database contains daily weather-station-based measurements of meteorological variables from over 90,000 stations globally. Approximately two thirds of the stations contain precitation measurements only. The other remaining core elements that are available at many stations are snowfall, snow depth, maximum temperature, and minimum temperature. A limited number of stations may also contain measurements of a wide range of elements, such as cloudiness, wind speed, soil temperature, etc. A full list of the available variables can be found at http://www1.ncdc.noaa.gov/pub/data/ghcn/daily/readme.txt. The remainder of this overview will focus on the daily minimum and maximum temperature data.
What are the key strengths of this data set?
The dataset contains direct measurements of near-surface air temperature (2m), with no interpolation or additional inference. For this reason, it is a crucial dataset for comparison and validation of other data types (satellite, reanalysis) that need to infer temperature via calibrations or the assimilated state of the atmosphere. Additionally, many individual station records go back a century or more, so the dataset can be used to assess changes in the climate over time.
What are the key limitations of this data set?
- Unequal spatial coverage: different regions of the world have different station densities based upon both the number of actual stations and whether or not the government has decided to freely share the data. The United States and Japan have relatively dense coverage. Coastal regions tend to have more stations that interior regions due to the location of population centers.
- Inhomogeneities: due to changes in various recording practices, there are inhomogeneities (non-climatic, or spurious) trends that are present in the dataset, three of which will be discussed in detail below. While many monthly datasets have been processed to remove the inhomogeneities, at the time of this writing there are no established homogenized daily datasets. The presence and influence of inhomogeneities are most well-documented in the United States (Menne et al., 2009) but can be assumed to be occurring to some extent in other parts of the world. These changes include (1) shifts in the time of observation, (2) switching of thermometer types, and (3) station moves.
(1) Shifts in time of observation
The GHCN-D database contains daily maximum and minimum temperature. The reason that these measurements are available rather than, e.g., average temperature is because of the invention of thermometers (such as Six's thermometer) that record the maximum and minimum temperature over a given period. With these thermometers, an observer can visit a thermometer once a day and record information about maximum and minimum temperatures over the prior 24 hours. Ideally, the thermometer would be checked at local midnight, but -- given that this is not a very convenient time for humans -- most thermometers are checked at some point during the day. Unfortunately, recording the data during the day tends to induce a bias in the measurements, which will be demonstrated by example. Imagine that the 24-hour max/min temperatures for a given weather station tend to be recorded at 5pm, which would have been fairly typical in 1970 (Vose et al., 2003). Between day 0 and day 1 at this station a cold front comes through, leading to a large drop in temperatures. If the temperature at 5:01pm on day 0 turns out to be warmer than the high temperature for day 1, then the maximum temperature for day 1 will be recorded as the late afternoon temperature from the previous day. In other words, the 24-hour maxima for this time period is not the same as the high temperature during day 1. Generalizing this example, it can be shown that recording temperature in the afternoon tends to produce warmer daily minimum and maximum temperatures than recording temperature in the morning. Furthermore, at least in the United States where recording practices are better documented, there has been a general shift from afternoon to morning measurement times, which induces a spurious cold trend in temperature.
(2) There has been a gradual shift from the use of Liquid in Glass thermometers such as the Six's thermometer to digital thermometers, called the Minimum-Maximum Temperature System, that are easier to read. Due to differences in the way that the thermometers are sited and sheltered, it appears that they have a bias with respect to each other. Based on a 20-year side-by-side study of the two thermometer types (Doesken, 2005), it appears that maximum temperatures are biased approximately 0.4--0.6 °C low with the new thermometers, and minimum temperatures are between 0.1°C too low or too high, where the range is a function of the season. Since the trend in the United States has been from Liquid in Glass to Minimum-Maximum Temperature System measurements, the switch would, on average, induce a spurious cold trend.
(3) The location of thermometer-containing weather stations has changed over time, even if the data is presented as representative of a single location. If there is a trend in land use, it is more likely to be towards urbanization, leading to concerns that there is a substantial urban heat island effect in temperature datasets. While the effect is not negligible in rapidly urbanizing countries like China, it is generally smaller than the 'true' warming induced by climatic changes (e.g. Jones et al., 2008).
- While not necessarily a key limitation, it is important to note that, while all temperature measurements are presented at 0.1 °C precision, this was not necessarily their original precision, or units. Rhines et al. (2015) present a 'precision-decoding' algorithm that is able to back out the true precision of the data. For example, if a temperature is presented as 5.5 °C , but the true precision of the measurement was 0.5 °C (e.g. measurements were only made in intervals of 0.5 °C ), then the uncertainty around the datum is 0.25 °C rather than 0.05°C .
What are the most common mistakes that users encounter when processing or interpreting these data?
Use of the data require a careful assessment of the biases that may be present due to changes in measurement practices. In general these errors will around an order of magnitude smaller than, e.g., a long-term trend, but should not be ignored.
What are some comparable data sets, if any?
- The US Historical Climatology Network contains a high-quality subset of the GHCND stations in the United States that have been determined to have sufficient metadata regarding measurement practices.
- the European Climate Assessment dataset, covering Europe and the Mediterranean
- For users specifically interested in daily extremes, the HadEX2 dataset contains daily-resolved values for 27 indices of extremes (Alexander et al., 2006).
How is uncertainty characterized in these data?
There are no quantitative uncertainty estimates in the data. However, measurements are presented with flags that indicate whether data is suspect.
How do I best compare these data with model output?
There is no perfect way to compare the point measurements available from weather stations to the gridded fields in climate models. One option is to statistically interpolate the station measurements to a climate model grid using techniques such as kriging. If one uses this approach, it is important to include uncertainty quantification in the interpolation method because of the highly variable station density. Recall, however, that the climate model output would still be expected to contain additional smoothness (even after interpolating the station measurements) because of the numerics of the model (e.g. diffusion).##
Cite this page
Acknowledgement of any material taken from or knowledge gained from this page is appreciated:
McKinnon, Karen & National Center for Atmospheric Research Staff (Eds). Last modified "The Climate Data Guide: GHCN-D: Global Historical climatology Network daily temperatures.” Retrieved from https://climatedataguide.ucar.edu/climate-data/ghcn-d-global-historical-climatology-network-daily-temperatures on 2025-05-11.
Citation of datasets is separate and should be done according to the data providers' instructions. If known to us, data citation instructions are given in the Data Access section, above.
Acknowledgement of the Climate Data Guide project is also appreciated:
Schneider, D. P., C. Deser, J. Fasullo, and K. E. Trenberth, 2013: Climate Data Guide Spurs Discovery and Understanding. Eos Trans. AGU, 94, 121–122, https://doi.org/10.1002/2013eo130001
Key Figures
Other Information
- Alexander, L.V. and others, Global observed changes in daily climate extremes of temperature and precipitation (2006). Journal of Geophysical Research: Atmospheres, 111:D05109
- Doesken, N.J., The National Weather Service MMTS (Maximum-minimum Temperature System)--20 years after (2005). Presented at 15th Applied Climatology Conference. Amer. Meteor. Soc., Savanna, GA
- Jones, P.D., D.H. Lister and Q. Li, Urbanization effects in large-scale temperature records, with an emphasis on China (2008). Journal of Geophysical Research: Atmospheres, 113:D16122
- Menne, M.J., C.N. Williams, Jr., and R.S. Vose, The U.S. Historical Climatology Network Monthly Temperature Data, version 2 (2009). Bulletin of the American Meteorological Society, 90:7 993--1007.
- Rhines, A., M.P. Tingley, K.A. McKinnon and P. Huybers, Decoding the precision of historical temperature observations (2015). Quaterly Journal of the Royal Meteorological Society 141:693 2923--2933.
- Vose, R.S., C.N. Williams, Jr., T.C. Peterson, T.R. Karl and D.R. Easterling, An evaluation of the time of observation bias adjustment in the U.S. Historical Climatology Network (2003). Geophysical Research Letters 30:20 CLM.